Apply the Three Criteria for Causation to This Claim, Given What You Know About the Methodology
Suggested Citation:"vi Research Methodology and Principles: Assessing Causality." National Academies of Sciences, Engineering, and Medicine. 2016. Commercial Motor Vehicle Driver Fatigue, Long-Term Health, and Highway Safety: Research Needs. Washington, DC: The National Academies Press. doi: 10.17226/21921.
×
6
Enquiry Methodology and Principles: Assessing Causality
One of the panel's master tasks was to provide information to the Federal Motor Carrier Safety Administration (FMCSA) on how the nigh upwards-to-date statistical methods could aid in the bureau's work. The theme of this affiliate is that methods from the relatively new subdiscipline of causal inference encompass several design and assay techniques that are helpful in separating out the bear upon of fatigue and other causal factors on crash take a chance and thereby determining the extent to which fatigue is causal.
A main question is the degree to which fatigue is a risk gene for highway crashes. Efforts have been made to assess the percentage of crashes, or fatal crashes, for which fatigue played a key role. Nonetheless, assessment of whether fatigue is a causal factor in a crash is extremely difficult and likely to suffer from substantial mistake for two reasons.
Showtime, the data collected can be of low quality. Biomarkers for fatigue that can provide an objective measurement after the fact are not bachelor. If drivers survive a crash and are asked whether they were drowsy, they may not know how drowsy they were, and even if they practise know, they have an incentive to minimize the extent of their drowsiness. In virtually cases, the police at the scene are charged with determining whether a chargeable law-breaking was committed; whether a traffic violation occurred; and whether specific conditions, such equally commuter fatigue, were or were not present. They must make this conclusion to the best of their abilities with limited information. Information technology is commonly accepted and under-
Suggested Commendation:"vi Inquiry Methodology and Principles: Assessing Causality." National Academies of Sciences, Applied science, and Medicine. 2016. Commercial Motor Vehicle Driver Fatigue, Long-Term Health, and Highway Safety: Inquiry Needs. Washington, DC: The National Academies Press. doi: x.17226/21921.
×
standable that police force underestimate the degree of drawn driving and its impact on crashes.
Police assessments, augmented by more than intense interviewing and other investigations, were used to determine factors contributing to crashes in such studies every bit the Big Truck Crash Causation Report (LTCCS) (see Chapter 5), in which the researchers attempted to determine the critical event (the outcome that immediately precipitated the crash) and the disquisitional reason for that event (the firsthand reason for the critical event) for each crash. To this stop, they tried to provide a relatively complete description of the conditions surrounding each crash. This approach is fundamentally different from that of calculating the per centum of crashes attributable to different causes. Neither approach is entirely satisfactory: in the LTCCS approach, the concept of a "critical reason" is not well defined since many factors can combine to cause a crash, with no individual gene being solely responsible, while in the other arroyo, the attributed percentages can sum to more than 100 percent.
Second, in addition to low-quality information, the fact that crashes oftentimes are the issue of the joint effects of a number of factors makes it difficult to determine whether fatigue contributed to a crash. Crashes can be due to factors associated with the driver (east.g., drowsiness, distractedness, anger); the vehicle (e.k., depth of tire tread, quality of brakes); the driving situation (e.one thousand., high traffic density, presence of road obstructions, icy road surfaces, low visibility, narrow lanes); and the policies of the carrier, including its arroyo to compensation and to scheduling. The and then-called Swiss cheese model of crash causation (Reason, 1990) posits that failures occur because of a combination of events at different layers of the phenomenon. Similarly, the and so-chosen Haddon Matrix (Runyan, 1998) looks at factors related to human, vehicle, and environmental attributes before, during, and after a crash. A synthetic matrix permits evaluation of the relative importance of different factors at dissimilar points in the crash sequence. These models admit that a traffic crash has a multitude of possible causes that may not function independently, resulting in a adequately complex causal structure. Therefore, understanding the role of an individual gene, such as fatigue, in causing a crash can be a claiming.
Given that crashes tin can have many causes, increases and decreases in crash frequency over time can exist due to changes in the frequency of whatsoever one of these causes. For instance, a harsher-than-usual winter might raise the frequency of crashes, everything else remaining constant. By ignoring such dynamics, one can exist misled about whether some initiative was or was not helpful in reducing crashes.
To draw proper inferences most crash causality, then, it is important to understand and control the various causal factors in making comparisons or assessments—including those outside of ane'southward interest, referred
Suggested Commendation:"6 Research Methodology and Principles: Assessing Causality." National Academies of Sciences, Engineering, and Medicine. 2016. Commercial Motor Vehicle Driver Fatigue, Long-Term Health, and Highway Safety: Enquiry Needs. Washington, DC: The National Academies Press. doi: 10.17226/21921.
×
to as confounding factors. Therefore, to assess the degree to which fatigue increases crash risk, one must account for the dynamics of the confounding factors, including whatever correlation between them and the causal factors of interest. This can be accomplished through design or analysis techniques.
A common design that limits the influence of confounding factors is the randomized controlled trial. For reasons given below, even so, most of the data collected in studies of motor carrier safety are observational, so methods are needed to help balance the touch on of confounders on comparisons of groups with and without a causal factor of interest. By using such methods, ane tin can better empathize the function of fatigued driving and therefore assist determine which policies should be implemented and warrant the allotment of resources to reduce crash risks due to fatigue.
The following sections begin past defining what is meant past causal event. This is followed past word of the inferences that are possible from information on crashes and the various kinds of standardization that might be used on crash counts. Next is an test of what tin can be determined through the use of randomized controlled trials and why they are not feasible for addressing many important questions. The advantages and disadvantages of data from observational studies—which are necessary for many topics in this field—are then reviewed. Included in this department is a clarification of techniques that tin be used at the design and assay stages to back up cartoon causal inferences from observational data and extrapolating such inferences to similar population groups.
DEFINITION OF CAUSAL Upshot
The definition of a causal effect applied in this affiliate is that of Rubin (see The netherlands, 1986). Assume that one is interested in the consequence of some treatment on some outcome of interest Y, and for simplicity assume that the treatment is dichotomous (in other words, handling or control). The potential outcome Y(J) is defined as the value of the upshot Y given treatment blazon J. And then the causal effect of the handling (as contrasted with the command) on Yi is divers as the difference in potential outcomes Yi (1) – Yi (0), defined as follows: a selected unit of measurement i (east.g., a person at a particular signal in time) given treatment Ji = 1 results in Yi (i), and the same selected unit given the control Ji = 0 results in Yi (0), with all other factors being held abiding. For instance, if what would accept happened to a subject field under a handling would have differed from what would take happened to the same subject at the same time nether control, and if no other factors for the field of study inverse, the difference between the treatment and the control is said to have caused the deviation. The problem when applying this definition is that for a given entity or situation, ane cannot
Suggested Citation:"6 Research Methodology and Principles: Assessing Causality." National Academies of Sciences, Engineering, and Medicine. 2016. Commercial Motor Vehicle Driver Fatigue, Long-Term Health, and Highway Safety: Research Needs. Washington, DC: The National Academies Printing. doi: ten.17226/21921.
×
discover what happens both when Ji = 0 and when Ji = one. One of these potential outcomes is unobserved, then one cannot estimate the unit-level causal effect. Given some assumptions well-nigh treatment constancy and intersubject independence, however, it is possible to estimate the average causal outcome across a population of entities or situations. To practice and then, since one is comparing situations in which J = i against those in which J = 0, one must utilize techniques that make it possible to assert that the units of analysis are as similar every bit possible with respect to the remaining causal factors.
Agreement causality is an important goal for policy analysis. If one understands what factors are causal and how they affect the effect of interest, 1 can then decide how the changes to causal factors even for a somewhat different situation from the one at manus volition impact the probability of various values for the outcome of involvement. If ane simply determines that a gene is associated with an result, however, it may be that the specific circumstances produced an apparent human relationship that was actually a by-product of confounding factors related to treatment and outcomes.
Cartoon INFERENCES AND STANDARDIZING CRASH COUNTS
Every bit one example of misreckoning and the challenges entailed in drawing causal inferences, it is mutual for those concerned with highway safety to plot crash counts past yr to assess whether road rubber is improving for some region. This type of analysis tin be misleading. For example, Figure six-1 shows a big decline in total fatalities in truck crashes between 2008 and 2009. It is mostly accepted that this decline was due to the substantial reduction in vehicle-miles traveled that resulted from the recession that started during that yr. However, it is also possible that the pass up was due in function to new safe engineering, improved brakes, improved structural integrity of the vehicles, or increased safety belt utilise. Thus, looking at a time series of raw crash counts alone cannot yield reliable inferences.
Every bit a first footstep in enabling better interpretation of the data, i could standardize the crash counts to account for the change in vehicle-miles traveled, referred to as exposure data. Thus an obvious initial idea is to apply vehicle-miles traveled as a denominator to compute crashes or fatal crashes per miles traveled. In some sense, exposure information are a type of confounding factor, because a truck or bus that is beingness driven less is less probable to exist involved in a crash. The lack of exposure data with which to create crash rates from the number of crashes is a trouble discussed below. Some other problem with normalizing crashes past dividing past vehicle-miles traveled is that the human relationship between the number of crashes and
Suggested Citation:"half-dozen Enquiry Methodology and Principles: Assessing Causality." National Academies of Sciences, Applied science, and Medicine. 2016. Commercial Motor Vehicle Driver Fatigue, Long-Term Wellness, and Highway Rubber: Research Needs. Washington, DC: The National Academies Printing. doi: 10.17226/21921.
×
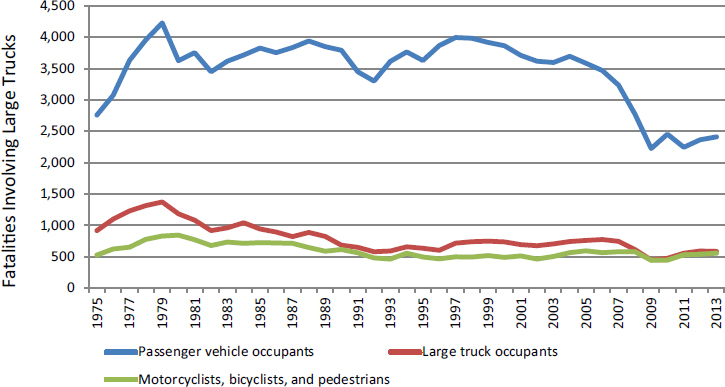
SOURCE: Insurance Establish for Highway Safety. Available: http://world wide web.iihs.org/iihs/topics/t/large-trucks/fatalityfacts/large-trucks [March 2016] based on the U.Due south. Section of Transportation'south Fatality Analysis Reporting System.
the amount of exposure might be nonlinear, as pointed out by Hauer (1995). This nonlinearity is probable due to traffic density equally an additional causal factor.
The idea of standardization can be extended. What if other factors could confound the comparison of fourth dimension periods? For example, suppose that in comparing two time periods, one finds that more than miles were traveled in i year under wet weather than in the other year? To accost this potential confounder, the information could be stratified into days with and without precipitation prior to standardizing past vehicle-miles traveled. Increasingly detailed stratifications tin can be considered if the data be for various factors. Nonetheless there are limits to which this can be done. At some point, one would have such an extensive stratification that there would likely be few or no crashes (and possibly even no vehicle-miles traveled) for many of the cells. To accost that effect, modeling assumptions could exist used in conjunction with various modeling approaches. For instance, one could assume that log [Pr(Crash)/(1 – Pr(Crash))] is a linear function of the stratifying factors, only this approach would rely on these assumptions being approximately valid.
An understanding of which factors are and are not causal and the extent to which they affect the upshot of interest is important in deciding on an appropriate standardization. Efforts at further standardization
Suggested Citation:"half dozen Research Methodology and Principles: Assessing Causality." National Academies of Sciences, Applied science, and Medicine. 2016. Commercial Motor Vehicle Driver Fatigue, Long-Term Health, and Highway Safety: Research Needs. Washington, DC: The National Academies Press. doi: x.17226/21921.
×
past other potential casual factors or potential confounders are as well constrained by the fact that police reports ofttimes include merely limited information on the driver, the vehicle, and the environs.
At present, the primary source of data for vehicle-miles traveled is the Federal Highway Assistants (FHWA). However, these data are too aggregate and lacking in specifics to exist used every bit denominators in producing crash rates for various kinds of drivers, trucks, and situations. Without exposure data, 1 might be able to separate collisions into those in which a factor was or was non nowadays (although doing so is difficult, run into Affiliate v). Nonetheless, since 1 would not know how much crash-free driving had occurred when that gene was and was not nowadays, i could non know whether the number of crashes when a cistron was nowadays was large or modest.
Office OF RANDOMIZED CONTROLLED TRIALS
Much of what is known most what makes a person drowsy, how being drowsy limits a one's performance, and what tin can be done to mitigate the effects of inadequate sleep derives from laboratory studies, which usually entail randomized controlled trials. For instance, studies have been carried out with volunteers to see how different degrees of sleep restriction affect response fourth dimension. For such an experiment, it is important for the various groups of participants to differ only with respect to the treatment of interest—for case, degree of slumber restriction—and for them not to differ systematically on whatever misreckoning factors. In randomized experiments, one minimizes the effects of confounders by randomly selecting units into treatment and command groups. As the sample size increases, the randomization tends to residue all confounders across the different groups. (That is, randomization causes confounders to exist uncorrelated with selection into treatment and control groups.) Traditional randomized controlled trials besides are unremarkably designed to have relatively homogeneous participants so that the treatment outcome tin more than easily be measured. This homogeneity is achieved by having restrictive entry criteria. Further, the treatment is normally constrained besides. While this homogeneity of participants and intervention improves assessment of the efficacy of the treatment outcome, it oftentimes limits the generalizability of the results.
In addition to restrictive entry criteria, stratification or matching is used to provide greater control over potential misreckoning characteristics. If such techniques are not used, the upshot tin can exist an imbalance between the handling and control groups on such characteristics, even with randomization into groups. For example, 1 could take more than elderly people in the treatment group than in the control group even with randomization. As the number of potentially causal factors increases, the opportunities for such imbalance also increase.
Suggested Commendation:"6 Research Methodology and Principles: Assessing Causality." National Academies of Sciences, Engineering, and Medicine. 2016. Commercial Motor Vehicle Driver Fatigue, Long-Term Health, and Highway Safety: Research Needs. Washington, DC: The National Academies Press. doi: 10.17226/21921.
×
Equally discussed beneath, for a number of topics involving field implementation, randomized controlled trials are not viable. 1 blazon of report, withal—the randomized encouragement pattern—provides some of the benefits of such trials but may be more feasible. In such studies, "participants may be randomly assigned to an opportunity or an encouragement to receive a specific treatment, but immune to choose whether to receive the treatment" (West et al., 2008, p. 1360). An example would be randomly selecting drivers to receive encouragement to be tested for sleep apnea and examining the effects on drivers' wellness (post-obit Kingdom of the netherlands, 1988). This type of design tin exist useful when the treatment of interest cannot be randomly assigned, but some other "encouragement" to receive the handling (such as a mailer or monetary incentive) can be randomly provided to groups of participants.
Before continuing, it is important to reiterate that electric current agreement of the influence of diverse factors on highway safety and on fatigue comes from a variety of sources, including laboratory tests, naturalistic driving studies, and crash data (run across Chapter 5). These diverse sources have advantages and disadvantages for addressing unlike aspects of the causal concatenation from various sources of sleep inadequacy, including violation of hours-of-service (HOS) regulations, to sleep deficiency, to lessened performance, to increased crash risk. I can recollect of these diverse sources of data every bit beingness plotted on a ii-dimensional graph of fidelity versus control. Typically, as ane gains allegiance—that is, correspondence with what happens in the field—one loses control over the various confounding factors. That is why it tin exist helpful to begin studies in the laboratory, only equally one gains knowledge, some field implementation is oft desirable. These latter studies volition often benefit from methods described in the next section for addressing the potential impacts of confounding factors.
OBSERVATIONAL STUDIES
Observational studies are basically surveys of what happened in the field (e.grand., on the route). If data were gathered from individuals who did and did not receive some intervention or treatment or did and did not engage in some beliefs, ane could compare any event of interest between those groups. Notwithstanding, any such comparison would suffer from a potential lack of comparability of the treatment and control groups on confounding factors. That is why techniques are needed to assist attain such balance after the fact. However, observational studies do take the advantage of collecting information that are directly representative of what happens in the field.
Further, such studies are generally feasible, which often is not the case for randomized controlled trials. For example, it is non possible to
Suggested Citation:"6 Research Methodology and Principles: Assessing Causality." National Academies of Sciences, Engineering, and Medicine. 2016. Commercial Motor Vehicle Commuter Fatigue, Long-Term Health, and Highway Rubber: Research Needs. Washington, DC: The National Academies Press. doi: 10.17226/21921.
×
randomize drivers to follow or non follow the HOS regulations. Such an experiment would obviously be unethical too as illegal. Similarly, drivers diagnosed with obstructive sleep apnea could not exist randomly divided into two groups, one treated with positive airway force per unit area (PAP) devices and the other non, to assess their crash risk on the highway. For nigh issues related to study of the function of fatigue in crashes, such random selection into handling and control groups is not feasible.
With a few exceptions, the data currently collected that are relevant to understanding the linkage between fatigue and crash frequency are observational (nonexperimental). Therefore, methods are needed for balancing the other causal factors between 2 groups that differ regarding some behavior or characteristic of interest so those other factors will not derange the estimates of differences in that cistron of interest. For example, not properly controlling for alcohol employ may lead to an overestimation of the furnishings associated with fatigue for nighttime driving. Thus without careful pattern and analysis, what one is estimating is not the issue of a certain factor on crash frequency but the combination of the effect of that factor and the difference between the treatment and control groups on some confounding factor(due south).
This point is illustrated past a study undertaken recently by FMCSA to determine whether the method of compensation of truck drivers is related to crash frequency. Here the type of compensation is the treatment, and crash frequency is the outcome of interest. A complexity is that carriers who chose a specific method for compensation might have other characteristics over- or underrepresented, such as their method for scheduling drivers or the type of roads on which they travel. Information technology is difficult to split the effect of the compensation approach from these other differences among carriers.
Regression Aligning
Instead of balancing these other causal factors by matching or stratifying, one might promise to correspond their issue on the issue of interest directly using a regression model. Here the dependent variable would be the event of involvement, the handling indicator would be the primary explanatory variable of interest, and the remaining causal factors would be boosted explanatory variables. The trouble with this technique is that the assumption that each of the explanatory variables (or a transformation of a variable) has a specific functional relationship with the consequence is a relatively strong assumption that is unlikely to be truthful. The farther apart are the values for the confounding factors for the treatment and control groups, the more 1 will take to rely on this supposition. (There are also nonparametric forms of regression in which the depen-
Suggested Citation:"6 Enquiry Methodology and Principles: Assessing Causality." National Academies of Sciences, Engineering, and Medicine. 2016. Commercial Motor Vehicle Driver Fatigue, Long-Term Health, and Highway Safety: Research Needs. Washington, DC: The National Academies Press. doi: ten.17226/21921.
×
dence on linearity is reduced, but some more than general assumptions all the same are made about how the outcome of involvement and the causal factors interact, for example, see Hill [2011].)
Blueprint Methods for Observational Information
This department describes 3 techniques used in conjunction with the collection of observational data in an attempt to derive some of the benefits of a randomized controlled trial by limiting the influence of confounding factors. Note that this is an illustrative, non a comprehensive list, and the terminology involved is not altogether standardized.
Cohort Study
A cohort of cases is selected and their causal factors measured equally role of an observational report database. So either the cases are followed prospectively to define their event status, or that cess is performed on historical records as part of a retrospective study.
Case-Control Report
To assess which factors do and practice not increase the adventure of crashes, one can identify drivers in an observational database who have recently been involved in crashes, and at the same time collect information on their characteristics for the causal factor(southward) of interest and for the misreckoning causal factors. Then, one identifies controls that match a given case for the confounding factors from among drivers in the database who have not been involved in contempo crashes. One next determines whether the causal factor(due south) of interest were or were not present more oftentimes in the cases than in the controls. An example might be to see whether fewer of those drivers recently involved in a crash relative to controls worked for a safety-conscious carrier, controlling for the driver'south torso mass index (BMI), experience, and other factors. If one did not lucifer the two groups of drivers on the confounding factors, this approach could produce poor inference, since the ii groups likely would differ in other respects, and some of those differences might be causal.
Example-Crossover Study
A instance-crossover design is used to answer the question: "Was the event of interest triggered by another occurrence that immediately preceded it?" (Maclure and Mittleman, 2000; Mittleman et al., 1995). Here, each case serves equally its own control. The design is analogous to a crossover experiment
Suggested Citation:"6 Research Methodology and Principles: Assessing Causality." National Academies of Sciences, Engineering, and Medicine. 2016. Commercial Motor Vehicle Commuter Fatigue, Long-Term Wellness, and Highway Safety: Research Needs. Washington, DC: The National Academies Press. doi: 10.17226/21921.
×
viewed retrospectively. An example might be a truck commuter who had been involved in a crash. One might examine whether the truck driver had texted in the previous hour and then come across whether the same driver had texted a week or a calendar month prior to the crash, and once again for several previous time periods. In that manner, 1 would obtain a measure of exposure to that beliefs close to the time of the crash and exposure more than by and large. (Of course, assessing whether a driver has texted is not e'er straightforward.)
Analysis Methods for Observational Data
This department describes some analytic methods that tin exist used to select subjects for analysis or to weight to achieve balance between a treatment and a control grouping on confounding factors.
Propensity Score Methods
One of the most common tools for estimating causal effects in nonexperimental studies is propensity score methods. These methods replicate a randomized experiment to the extent possible by forming treatment and comparison groups that are similar with respect to the observed confounders. Thus, for case, propensity scores would allow one to compare PAP device users and nonusers who appear to exist similar on their prestudy health behaviors, conditions, and driving routines. The propensity score summarizes the values for the confounders into the propensity score, defined equally the probability of receiving treatment as a function of the covariates. The groups are so "equated" (or "balanced") through the use of propensity score weighting, subclassification, or matching. (For details on these approaches, see Rosenbaum and Rubin [1983]; Rubin [1997]; and Stuart [2010]. For an awarding of this method to highway safety, see Wood et al. [2015].)
Propensity score methods utilize a model every bit does regression aligning, just not in the same way. Propensity score methods have two features that provide an reward relative to regression aligning: (ane) they involve examining whether there is a lack of overlap in the covariate distribution betwixt the handling and control groups, and whether there are sure values of the covariates at which any inferences about handling effects would rely on extrapolation; and (two) they split up the design from the assay and allow for a "blinded approach" in the sense that ane can work hard to fit the propensity score model and conduct the matching, weighting, or subclassification (and assess how well they worked in terms of balancing the covariates) without looking at the consequence.
Both propensity score methods and regression adjustment rely on
Suggested Citation:"half dozen Enquiry Methodology and Principles: Assessing Causality." National Academies of Sciences, Engineering, and Medicine. 2016. Commercial Motor Vehicle Driver Fatigue, Long-Term Health, and Highway Safety: Enquiry Needs. Washington, DC: The National Academies Press. doi: 10.17226/21921.
×
the supposition that at that place are no unmeasured confounding factors. Techniques described below, such as instrumental variables and regression aperture, are ways of attempting to deal with potential unmeasured confounding. The assumption of no unmeasured confounders cannot be tested, but one can employ sensitivity analyses to assess how sensitive the results are to violations of that assumption (for details, meet Hsu and Pocket-size [2013]; Liu et al. [2013]; and Rosenbaum [2005]).
Marginal Structural Models
Propensity score methods are easiest to use when there is a relatively elementary and straightforward time ordering: (1) a point-in-time treatment with covariates measured before treatment, (2) a treatment administered at a single point in time, and (3) outcomes measured afterwards treatment. For more complex settings with fourth dimension-varying covariates and treatments, a generalization of propensity score weighting—marginal structural models—can be used (for details, see Cole and Hernan [2008] and Robins et al. [2000]). These approaches are useful if, for example, 1 has data on drivers' PAP use over fourth dimension, likewise every bit on measures of their slumber or wellness status over time, and ane wants to adjust for the misreckoning of wellness behaviors over time.
The basic idea of the marginal structural model is to weight each observation to create a pseudopopulation in which the exposure is independent of the measured confounders. In such a pseudopopulation, i can backslide the upshot on the exposure using a conventional regression model that does non include the measured confounders equally covariates. The pseudopopulation is created by weighting an observation at time t by the inverse of the probability of the observation's being exposed at time t, that is, past weighting past the inverse of the propensity score at time t.
Equally noted, marginal structural modeling can be thought of as a generalization of propensity score weighting to multiple fourth dimension points. To describe the method informally, at each fourth dimension point, the grouping receiving the intervention (e.1000., those receiving PAP treatment at that time point) is weighted to look like to the comparison grouping (those not receiving PAP treatment at that time point) on the footing of the confounders measured upwardly to that time point. (These confounders can include factors, such as sleep quality, that may accept been affected by a given individual'due south prior PAP use). As in propensity scoring, the weights are constructed as the estimated inverse of probability of receiving the treatment at that point in time. Then those individuals who take a large chance of receiving the treatment are given a smaller weight, and similarly for the comparison group, which results in the groups being much more comparable. The causal effects are then estimated by running a weighted model of the outcome
Suggested Citation:"6 Research Methodology and Principles: Assessing Causality." National Academies of Sciences, Engineering, and Medicine. 2016. Commercial Motor Vehicle Driver Fatigue, Long-Term Wellness, and Highway Safety: Research Needs. Washington, DC: The National Academies Press. doi: 10.17226/21921.
×
of involvement (eastward.chiliad., crash charge per unit) as a role of the exposure of involvement (e.g., indicator of PAP use). (The measured confounders are not included in that model of the consequence; this is known as the "structural" model).
Apply of Multiple Control Groups
Using multiple control groups is a way of checking for potential biases in an observational report (Rosenbaum, 1987; Stuart and Rubin, 2008). An observational study volition be biased if the control group differs from the treatment group in ways other than non receiving the handling. In some settings, ane can choose two or more control groups that may accept different potential biases (i.eastward., may differ from the treatment group in dissimilar ways). For example, if one wanted to written report the annual change in crash rates due to truck drivers' having increased their BMI by more than 5 points in the previous twelvemonth to a full of more than 30, such truck drivers might be compared with drivers who had BMIs that had not changed by more than 5 points and still had BMIs under xxx, and the same for bus drivers. If the results of these comparisons were similar (or followed an expected ordering), the study findings would be strengthened. Thus, for example, the findings would exist stronger if ane of the 2 command groups differed in that one had a college expected level of unmeasured confounders than the treatment group had, while the other control grouping had a lower expected level, and the results were consequent with that agreement. If, even so, i believed that there were no unmeasured confounders, but the command groups differed significantly from each other (then that the comparisons of the treatment and control groups differed significantly), that conventionalities would take to be wrong, since the difference in control groups could not be due to the treatment. (This is referred to as bracketing and is described in Rosenbaum [2002, Ch. eight].)
Instrumental Variables
Another common technique for use with observational information is instrumental variables. This arroyo relies on finding some "instrument" that is related to the treatment of interest (e.g., the use of some fatigue alerting applied science) merely does non directly affect the upshot of involvement (e.thousand., crash rates). In the fatigue alerting example, such an instrumental variable could be the indicator of a health insurance program that provides free fatigue alerting devices to drivers. Drivers in that plan could be compared with those non in the plan, under the supposition that the program might increment the likelihood of drivers using such a device merely would non directly affect their crash risk, except through whether they used the device. The advantage here is that there would be a good risk that the drivers who did
Suggested Citation:"6 Research Methodology and Principles: Assessing Causality." National Academies of Sciences, Engineering, and Medicine. 2016. Commercial Motor Vehicle Driver Fatigue, Long-Term Wellness, and Highway Safe: Research Needs. Washington, DC: The National Academies Press. doi: 10.17226/21921.
×
and did not receive the gratis devices would be relatively comparable (perhaps depending on additional entry criteria for the plan).
The introduction of such instrumental variables tin can be a useful design, but it can be hard to identify an appropriate instrumental variable that is related strongly enough to the treatment of interest and does not have a direct effect on the issue(s) of interest. 1 potentially useful arroyo to addressing this issue is use of an encouragement design (similar to that discussed above), in which encouragement to receive the treatment of interest is randomized. Using PAP devices as an example, a randomly selected group of drivers would exist given some kind of encouragement to use the devices. This randomized encouragement could then be used as an instrumental variable for receiving and using the device, making information technology possible to examine, for instance, the effects of PAP utilize on crash rates. (For more than examples of and details on instrumental variables, come across Angrist et al. [1996]; Baiocchi et al. [2010]; Hernán and Robins [2006]; and New-firm and McClellan [1998].)
Regression Aperture
Regression discontinuity can be a useful design when an intervention is administered only for those exceeding some threshold quantity. For instance, everyone with a hypopnea score above some threshold would receive a PAP device, and those below the threshold would not. The analysis then would compare individuals just above and just below the threshold, with the idea that they are probable quite similar to one another except that some had admission to the handling of interest while others did not. Bloom (2012) provides a adept overview of these designs.
Interrupted Fourth dimension Series
Interrupted time series is a useful approach for estimating the effects of a detached change in policy (or law) at a given time (come across, e.g., Biglan et al., 2000). The analysis compares the outcomes observed afterwards the change with what would have been expected had the change not taken identify, using data from the period before the change to predict that counterfactual.
One useful aspect of this approach is that it tin can be carried out with information on just a single unit (east.m., one state that changed its police force), with repeated observations earlier and afterward the change. Withal, the design is stronger when there are besides comparing units that did not implement the change (such as a state with the same policy that did not change it), which tin can aid provide data on the temporal trends in the absence of the modify. This could be useful, for instance, for examining the consequence of a change in a company health plan if data also were available from a visitor that did
Suggested Citation:"6 Research Methodology and Principles: Assessing Causality." National Academies of Sciences, Engineering, and Medicine. 2016. Commercial Motor Vehicle Driver Fatigue, Long-Term Health, and Highway Safety: Research Needs. Washington, DC: The National Academies Printing. doi: 10.17226/21921.
×
not brand the change at that fourth dimension. These designs, with comparison subjects, are known every bit "comparative interrupted time series" designs.
A special instance of comparative interrupted times series is difference-in-difference estimation, which is basically a comparative interrupted time series pattern with only two points, before and after the alter. This approach compares the differences before and after the change betwixt two groups, 1 that did and one that did not experience the change. This approach enables controlling for secular changes that would accept taken place in the absence of the change of interest, as well every bit differences between the groups that do not change over time. (A good reference for these designs is Meyer [1995].)
Sensitivity Assay
For propensity score approaches, instrumental variable analyses, and many of the other techniques described hither, it is useful to decide the robustness of one's inference through the use of sensitivity assay. As noted above, one of the key assumptions of propensity score matching is that bias from unobservable covariates tin be ignored. If one could model the effect of unobserved covariates, one could test this assumption by calculating the divergence between estimated handling furnishings—after controlling for observed covariates and the effect of unobserved covariates. If the estimated handling effect were essentially erased past unobservable covariates, one could conclude that the treatment effect was due to the bias from unobservable covariates and was not a truthful treatment effect. However, testing the assumption is impossible because researchers exercise non have information on unobservable covariates. Therefore, a researcher would need to obtain a proxy for the bias from unobserved covariates, which would require a detailed understanding of the miracle being researched. As a result, sensitivity analysis procedures involve examining how much unmeasured confounding would need to be present to modify the qualitative conclusions reached and and so trying to make up one's mind whether that degree of confounding is plausible. (For details, see Hsu and Pocket-sized [2013]; Liu et al. [2013]; and Rosenbaum [2005].)
Generalizing Findings from Observational Studies to a Different Population
Often information technology is necessary to draw inferences for a population for which directly relevant inquiry has non been carried out. A key instance in the present context is drawing inferences about commercial motor vehicle drivers when the relevant research is for passenger car drivers. When is it safe to make such an extrapolation?
Suggested Citation:"6 Enquiry Methodology and Principles: Assessing Causality." National Academies of Sciences, Engineering, and Medicine. 2016. Commercial Motor Vehicle Driver Fatigue, Long-Term Health, and Highway Safety: Inquiry Needs. Washington, DC: The National Academies Press. doi: 10.17226/21921.
×
In this case, ane needs outset to assess internal validity for the population on which the relevant report was done, so assess the generalizability of the findings to another population of interest. The internal validity question involves the force of the causally relevant inference that can exist fatigued near a given enquiry question for the population and treatment studied (which may differ from the population and treatment of interest). The answer volition naturally depend on the report blueprint and assay plans. Different study designs take different implications regarding what tin can be concluded. The second issue is the generalizability of the findings. The promise is that the findings tin be translated to the assistants of the same or a closely related treatment for a similar population.
Criteria for determining the degree to which a study enables causal inference have been considered for many decades. In the area of medical and epidemiologic studies, one well-recognized set of criteria was avant-garde by Loma (1965). These criteria have evolved over time, and a summary of their modern interpretation is as follows:
- Forcefulness of association between the treatment and the outcome: The clan must be stiff plenty to support causal inference.
- Temporal relationship: The treatment must precede the outcomes.
- Consistency: The association between handling and outcomes must be consistent over multiple observations among unlike populations in unlike environments.
- Theoretical plausibility: There must be a scientific statement for the posited touch of the handling on the outcome.
- Coherence: The pattern of associations must be in harmony with existing knowledge of how the treatment should behave if it has an effect.
- Specificity: A theory exists for how the handling affects the outcome of interest that predicts that the handling will exist associated with that outcome in sure populations but not associated (or less associated) with other outcomes and in other populations, and the observed associations are consistent with this theory. Furthermore, alternative theories do not brand this same set up of predictions (Melt, 1991; Rosenbaum, 2002).
- Dose-response relationship: Greater exposure to the risk gene is associated with an increase in the event (or a decrease if the treatment has a negative effect on the outcome).
- Experimental evidence: Any related research will brand the causal inference more plausible.
- Analogy: Sometimes the findings tin be extrapolated from another coordinating question.
Suggested Commendation:"6 Research Methodology and Principles: Assessing Causality." National Academies of Sciences, Engineering, and Medicine. 2016. Commercial Motor Vehicle Driver Fatigue, Long-Term Health, and Highway Safety: Research Needs. Washington, DC: The National Academies Press. doi: 10.17226/21921.
×
The panel suggests an additional criterion—the elimination of alternative explanations for observed associations—to be key in helping to found a causal relationship.
While criteria for establishing causal relationships accept evolved over fourth dimension, the principles articulated by Loma are notwithstanding valid. The panel wishes to emphasize the criteria of consistency, theoretical plausibility, coherence, and experimental evidence, which back up the point that causal inference often is non the event of a unmarried study simply of a process in which evidence accumulates from multiple sources, and support for alternative explanations is eliminated. As described in this chapter, the past xxx years besides have seen many advances regarding methods for estimating the effects of "causes" or interventions in nonexperimental settings.
There is value, then, in using a diverseness of approaches to meliorate empathise the arguments that can be fabricated as to whether a treatment or an intervention has an effect. Doing and so makes it possible to gain causally relevant knowledge from the collection of relevant studies so as to obtain the all-time possible understanding of the underlying miracle.
A expert case of how causality tin can be established primarily through observational studies is the relationship between cigarette smoking and lung cancer. In the 1950s, Doll and Hill (1950) and others carried out a number of observational studies on the association between cigarette smoking and lung cancer. These studies had the usual limitations and potential for confounding factors common to such studies. Still stiff associations were found beyond multiple populations and settings, and this clan also was shown to be monotonically related to the corporeality of smoking (meet Loma's criterion on the dose-response human relationship above). Some, however, including R.A. Fisher, proposed an alternative explanation: that at that place existed a factor that increased both the likelihood a person would utilise tobacco and the run a risk of contracting lung cancer, such as a genetic variant that made a person more likely to fume and more likely to contract lung cancer through contained mechanisms. This culling hypothesis was placed in doubt by a sensitivity analysis showing that if such a gene existed, it would need to take an association with smoking at least as keen as the observed association between smoking and lung cancer, and the proposed factors, such as genetic variants, were unlikely to accept such a strong association with smoking. Other alternative hypotheses were systematically rejected (see Gail, 1996). Even though a randomized controlled study of tobacco employ was clearly infeasible, it became clear through the diversity of available studies that supported the hypothesis and failed to support the rival hypotheses that cigarettes were a causal cistron for lung cancer.
The spectrum of observational study types includes retrospective cohort and case-command studies, prospective studies, and various types of
Suggested Commendation:"6 Research Methodology and Principles: Assessing Causality." National Academies of Sciences, Engineering, and Medicine. 2016. Commercial Motor Vehicle Driver Fatigue, Long-Term Wellness, and Highway Safety: Enquiry Needs. Washington, DC: The National Academies Printing. doi: x.17226/21921.
×
designs based on observational information, described by Shadish and colleagues (2002). These techniques, and additional ideas described here, have been applied in a number of policy areas and can exist used to reduce the opportunity for misreckoning factors to influence outcomes when a written report does not have a randomized controlled blueprint.
Once treatment efficacy has been addressed through a causal understanding of the phenomenon, one is left with the question of the generalizability of the findings from the available studies to other populations and interventions. What one would similar is to have a sufficiently articulate understanding of the science underlying a finding of treatment efficacy that one tin transfer the finding to the assistants of the same or a closely related handling for different populations. For an excellent discussion of this outcome, see Pearl and Bareinboim (2011).
Suggested Citation:"6 Enquiry Methodology and Principles: Assessing Causality." National Academies of Sciences, Engineering, and Medicine. 2016. Commercial Motor Vehicle Driver Fatigue, Long-Term Wellness, and Highway Safety: Research Needs. Washington, DC: The National Academies Press. doi: 10.17226/21921.
×
This folio intentionally left blank.
knetespromfonston.blogspot.com
Source: https://www.nap.edu/read/21921/chapter/10
0 Response to "Apply the Three Criteria for Causation to This Claim, Given What You Know About the Methodology"
Post a Comment